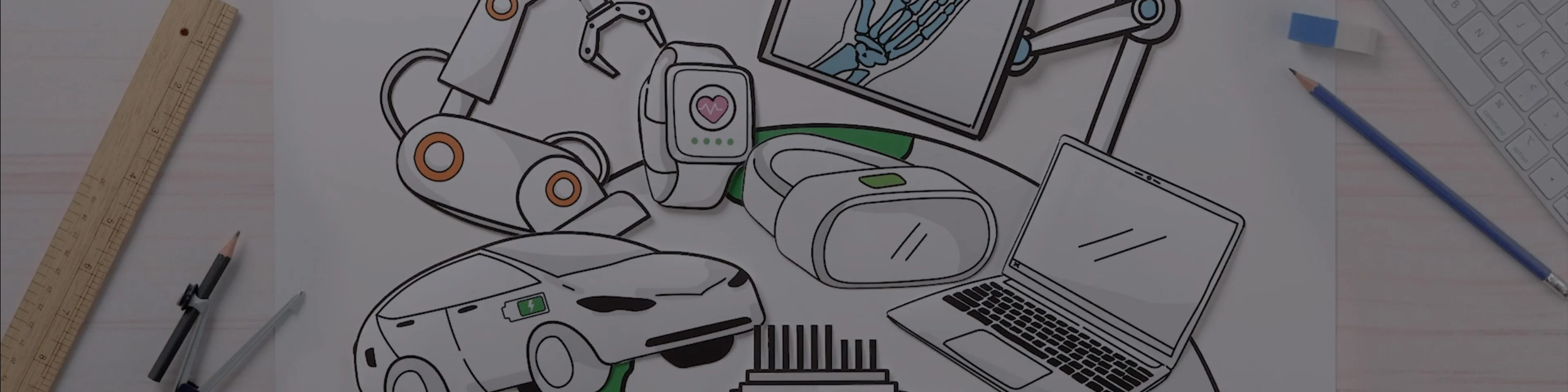
Blog
Thermal resistance prediction model for IC packaging optimization and design cycle reduction
Guan-Wei Chen; Yan-Cheng Lin; Chung-Hsiang Hsu; Tang-Yuan Chen; Chen-Chao Wang; Chin-Pin Hung
The development of highly reliable packaging products is paramount, as accurately predicting the thermal resistance values (Theta JA, JB, JC) of IC packages is crucial for calculating maximum power dissipation and mitigating self-heating effects. In this paper, we focus on the prediction and analysis of thermal resistance in packaged products. Traditionally, finite element methods (FEM) are used for simulations to predict thermal resistance. However, these simulations often employ idealized assumptions that may not align with real-world conditions. Furthermore, traditional simulation methods require substantial memory space and high-performance hardware, leading to significantly increased computation times as the complexity of the simulation increases, thereby extending the product verification timeline. Machine learning methods can effectively improve operational speed and reduce costs. This research utilizes datasets generated from past FEM simulations, testing various machine learning models, among which the Artificial Neural Network (ANN) models, a deep learning approach, yielded the best results. These models were then primarily employed for predicting the characteristics of packaged components. The findings indicate that the predicted results closely align with the FEM simulation data, with the percentage error in thermal resistance for QFN packages being less than 5%, thereby achieving structural optimization of packaged products and shortening the design cycle. Additionally, a user interface platform was developed to enhance research efficiency significantly. Researchers simply need to select the desired packaging technology product and enter the structural parameters to generate predictive results and visual charts, making it accessible even for operators lacking programming skills. Ultimately, the goal is to achieve visual structural optimization and shorten the design cycle for IC packaged products, serving as a basis for future structural studies of products.
From the model prediction results, the ANN model can accurately predict changes in thermal resistance values, with an error rate of less than 5%. At the same time, given changes in thermal resistance values under other external conditions, it can reduce the error rate and achieve structural optimization. In addition, from the perspective of the importance of the ANN model, the Length parameter of DIE determines most of the ANN model prediction capabilities. The main change in the thermal resistance value is determined by the Length parameter of DIE, which shortens the structure design cycle of future QFN packaging products.
Published in: 2024 IEEE 74th Electronic Components and Technology Conference (ECTC)
Popular Blog Posts
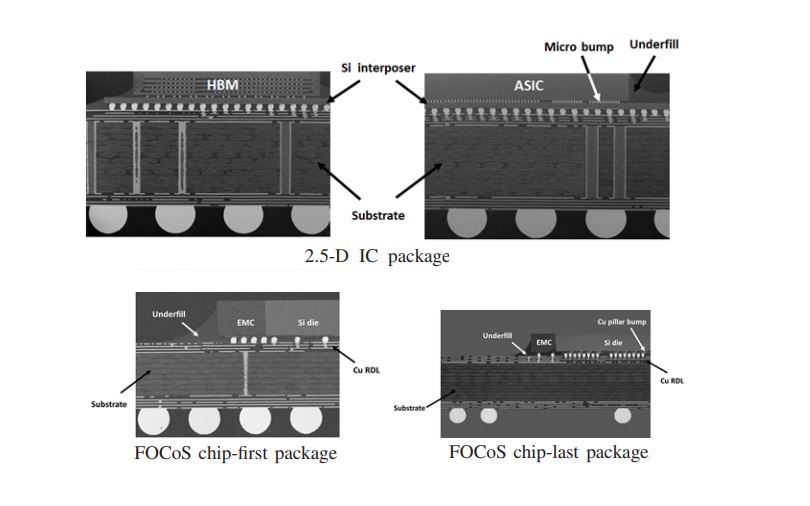
Thermal and Mechanical Characterization of 2.5D and Fan-Out Chip on Substrate Chip-First and Chip-Last Packages
2022 / 01 / 25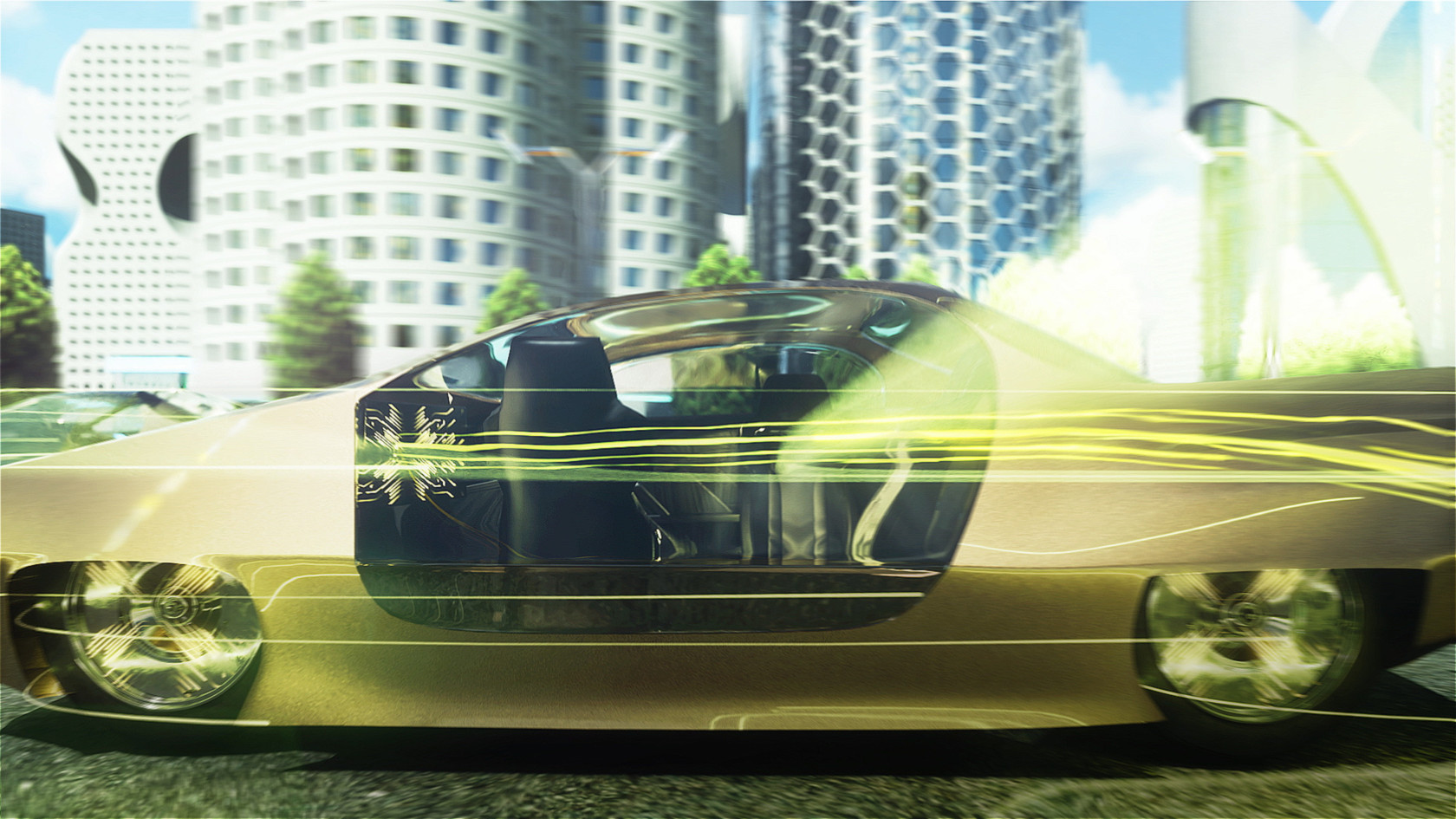
Innovation and Collaboration in Power Module Packaging: A Thermal Perspective
2025 / 03 / 03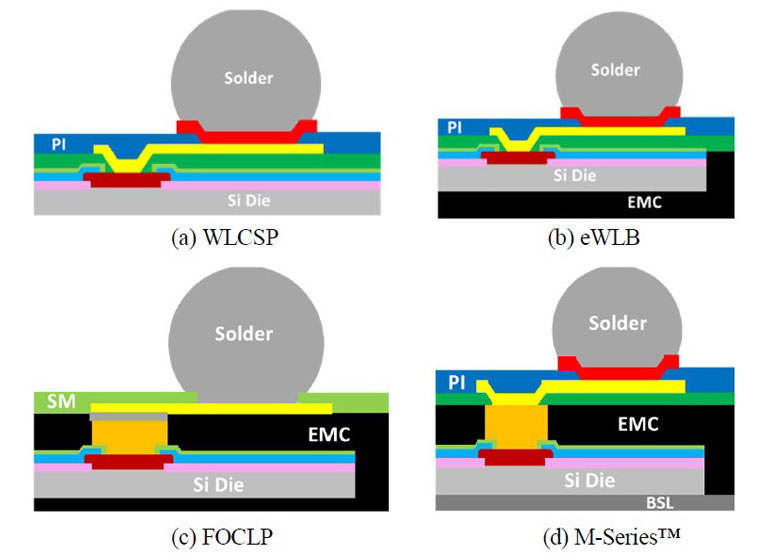
Comparative Study on Mechanical and Thermal Performance of eWLB, M-Series™ and Fan-out Chip Last Packages
2020 / 11 / 16