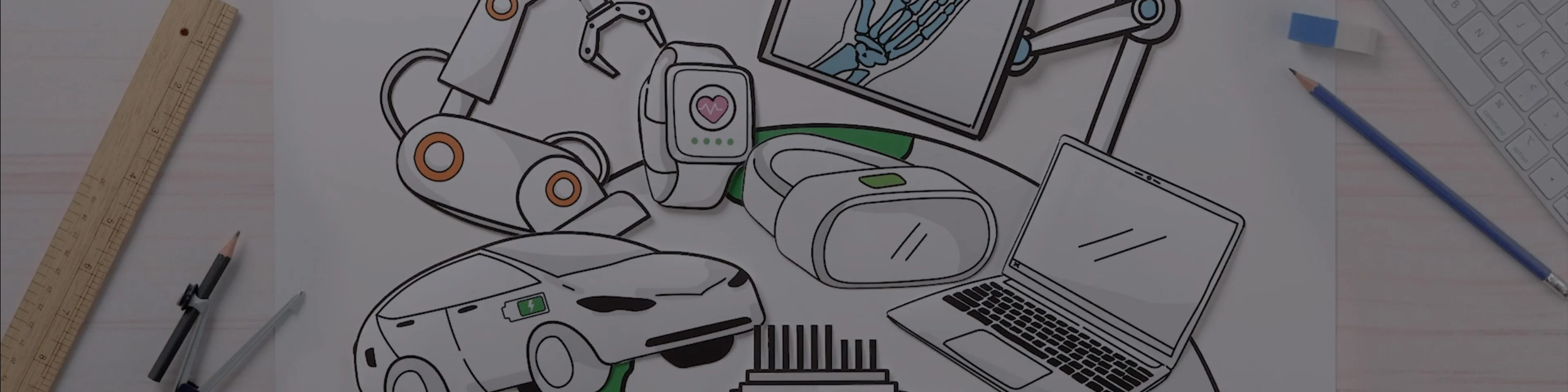
Blog
A Precision Enhancement Deep Learning Framework for Package Substrate Defect Detection
Yi-Chung Hsu; Wong-Shian Huang
In the manufacturing industry, producing a large quantity of highquality products every day is the top priority. Recently, the integration of Automated Optical Inspection (AOI) equipment and Deep Neural Network (DNN) defect image predictions has demonstrated a significant reduction in manual effort required for defect inspection in manufacturing industrial applications. However, during substrate manufacturing, changes in in-process conditions can lead to unexpected variations in defects, such as combinations of multiple defects or anomalies that humans are not familiar with. Consequently, mainstream DNN-based object detection (OD) methods have yet to achieve the desired accuracy for detecting these defects images in production line, and hence human visual inspections remain essential.
In this paper, we propose a defect detection framework to both reduce the need for human examination and maintain the high quality of normal products for downstream processes. This method integrates three DNN methods: OD, anomaly detection (AD), and auxiliary classification (AC). OD constructs hyperplanes for bounding-box objects and classes. AD measures distances between embedding vectors trained from normal samples to detect anomalies. AC operates by centering the object proposed by OD within a cropped image and re-judging the object’s class, which can refine OD’s anchor-based training. We conducted an ASE manufacturing dataset experiment, and our method reduced human examination by 28%, with only a 2.8% underkill rate. Combining OD and AC achieved 93.21% defect classification accuracy, surpassing mainstream accuracy 89.74%. With the assistance of our new framework, we are able to simultaneously reduce the manual efforts for examination and increase the accuracy of defect classification. When applied in MFG production lines, this technology can surely assist our factory operation, enabling us to better meet a smart factory requirements.
Published in: 2023 18th International Microsystems, Packaging, Assembly and Circuits Technology Conference (IMPACT)